Assessing the Total Addressable Market is a key element of every
business plan. TAM should, however, not be confused with the actual current
market size as I have explained in my previous post called
How To Size a Market.
In short, TAM represents the maximal
potential market. So, if everyone who could possibly buy your product bought
it, times the price of each unit - that is the total addressable market.
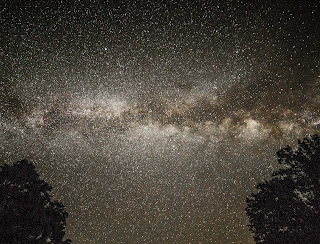 |
Think big when calculating your TAM |
TAM calculations are in general not concerned with your ability
to execute and with the market’s readiness. That means, when calculating your
TAM, you are not worried about your geographical presence, your competitive win
rate, your ability to avoid discounting, the strength of your brand, or your
products’ maturity and quality. All those factors (and more) ultimately reduce
the TAM down to what is your current revenue.
Between those two data points lie other metrics that are
sometimes used such as Serviceable Available Market (SAM) or Serviceable
Obtainable Market (SOM).
Those metrics are basically derived by applying some of the execution
constraints on the TAM like a set of filters. While interesting, those metrics
become very subjective and specific to any organization. For instance, your
absence in certain geographies reduces your SAM but that absence is a result of
your recent decision-making and it may or may not be easily revisited (i.e. by
entering geographies such as Japan, China, or Africa). That’s why TAM is the
most common metric because it avoids all such nuances and recent strategic and
tactical business decisions.
Finding TAM
So, how do you determine your TAM? First, search around and see
whether it already exists. In established markets, one of your competitors, an
industry analyst, or an investment bank might have already published a TAM,
whether or not they disclose how they came up with it. If you find such data
point, it’s your lucky day. Senior management and investors love to quote
Gartner or Goldman Sachs and their numbers are hardly ever questioned.
There are a few other possible data sources including large
system integrators (Deloitte, Accenture, etc.) as well as some of the online
collections of useful and less useful statistics such as
eMarketer
and
Statista. Obviously, publications such as
The Economist, The Wall Street Journal, and Business Insider also frequently
quote useful data points. It’s a good habit to collect the articles with
relevant data points for when you might need them.
But, let’s face it, you are probably reading this article because
you have not been successful finding your market’s TAM and you are stuck. Well,
if you can’t find it, you have to calculate it.
Calculating TAM
There are multiple methods of calculating TAM. Each one involves
certain judgment calls and educated estimates. This is key – to calculate TAM,
you will have to rely on your market expertise and make some estimates. Just
like I discussed in my previous blog post on calculating the market size, your
educated estimates will be much better than no data at all. After all, that’s
exactly what the analysts at Gartner, Deloitte, and Goldman Sachs do.
The three methods I will discuss here are:
1. Bottom
Up Calculation
The bottom up method is based on your
licensing model and estimates the maximum number of licenses available in the
world.
2. Top
Down Estimate
The top-down method is based on the
share of valet from the overall worldwide spending in a given sector.
3. Economic
Impact Estimate
This method is based on the estimate of
the economic impact of your product and what companies might be willing to
spend to capture that benefit.
I’m sure that there are other methods out there but these three I
have found most practical. So, let’s take a closer look:
Bottom-Up Calculation
This is my favorite method of estimating the TAM because it tends
to be the most accurate and relies on data that is hopefully available with
some level of accuracy. Simply put, this method counts the maximum number of
licenses that you could possibly sell. If your licensing is by household, you
count the number of households. If you are licensing by sales person, you count
the number of sales people. And if your licensing is based on number of wind
turbines, you count all the wind turbines out there.
Let’s take a specific example. Let’s say that you are
manufacturing a black box device for aircraft. Your licensing is basically per
aircraft and hence you need to the data on the number of aircraft in the world.
That data exists – it won’t take you long to find a number of data sources
providing the annual production for key manufacturers, the active fleet for
each airline, etc. You as the experts in this space should know those data
sources and be able to assess their validity.
Now, that you have the maximum possible number of licenses, you
multiply it by the price of your unit and voila, that’s your TAM. Of course you
can get more granular. Let’s say that your product is only for commercial
aircraft and not for private jets. You can calculate your TAM based on that. Or
maybe the long haul jets require two units – you can adjust your TAM
accordingly.
But remember, as you are adjusting your TAM to fit your specific product,
you might cross the line from TAM to SAM or SOM because some of these filters
are based on your business decisions that have reduced your addressable market.
For example – not selling to private jets might be a smart GTM focus but they
need your black boxes just as much as the commercial jets and your TAM should
reflect that. While it is important to put some boundaries around your
company’s or product’s opportunity, don’t restrict that opportunity based on
tactical thinking.
The challenge of this method is that the number of possible
licenses might not exist or that it is not precise enough. Let’s say you
license by number of sales people, but your product is only relevant to those
who are on the road every day. Or maybe it’s only for sales people selling
insurance. Finding that data may prove much more difficult. Still, there are
many sources worth checking out:
Gallup,
Nielsen,
Pew Research
Center,
US Bureau of Economic Analysis,
US
Bureau of Labor Statistics,
US Census Bureau,
Data.gov,
Reuters Data Dive, and many others.
Another way to get to relevant data is your own customer base.
Let’s say that you have a product licensed for your customers’ IT helpdesk and
so you need to know their number of IT helpdesk workers in the world. Analyzing
your own customer data, you might be able to determine that your customers have
on average 8% of their employees in IT and out of those, 25% work the helpdesk.
Knowing this ratio is extremely useful and if you have at least a few hundred
customers, it is very accurate.
With that ratio, you will need to establish the employee
population in your target market. If you sell to Financial Services, it’s easy
to find out that there are 6.3 million workers in that sector. If 2% of them work
the IT helpdesk, you have your TAM.
If you can’t find the employee population in your target market,
you can get it from the number of companies and their respective employee
numbers. That data is available from data sources such as Dun & Bradstreet,
Lightning Data (Salesforce), LinkedIn, NAICS.com, and others.
A similar process works for other licensing models - number of
vehicles, terabytes of data, or megawatts of energy produced. Sure, sometimes
you may need to estimate some of the data points. This is where your own
expertise comes in. It’s OK to estimate but always, document your estimates and
data sources. That way, anyone who doesn’t agree with any of your decisions can
follow your logic and adjust accordingly if they think they know better.
Top-Down Estimate
The top down estimate is based on the share-of-valet calculation
for your product. The basic idea is that from a macro-economical standpoint, there
is a finite amount of money spent on certain goods or services. That spend is
often well documented as several analyst firms publish the total annual spend
on markets such as IT, retail, travel, advertising, etc.
I’ll stick to the IT sector, since this is a blog on technology
and that’s what I know. Here, firms like Gardner, Forrester and IDC regularly publish
data on the worldwide market spend for IT technologies. Let’s take Gardner –
they forecast it for the next five years in their quarterly IT Spending
Forecast. That number is not going to grow because of your product, no matter
how amazing it is. That means, you need to take some of the share of valet from
the all other products. In other words, you’ll need to convince the IT buyers
to spend some of their precious budget on your product at the cost of all the
other IT spend. You need to take over some share of their valet.
The Gardner forecast gives you some amount of granularity, using
a two-layer taxonomy for software and providing the data for each of the
categories and sub-categories. For example, under CRM, Gardner forecasts the
following sub-categories: Customer Service and Support, Digital Commerce
Platforms, Marketing, and Sales. This is very helpful to further narrow your
available share-of valet down to the respective subcategory.
Your product will likely occupy an even narrower category and you
will need to estimate the share your category will take from the Gardner
taxonomy. Let’s say your product is a CPQ solution (Configure, Price, Quote),
which clearly falls under the Sales sub-category under CRM. Now, the good news
is that because this is a category recognized by Gardner (with its own Magic
Quadrant) there is likely more data available, including the current market
size and maybe some breakdowns by geography and vendors. Gardner doesn’t
publish this data but they track it and they will share it if you have the
right subscription.
If that data doesn’t exist, you need to estimate. List all of the
solution types in a given sub-category and assign them percentages based on
your best judgment. Again, your educated estimate is going to be better than no
data at all. But in general, this method is more useful for market sizing and
forecasting than to estimate the TAM. Still, having the understanding of the
overall market spend and its taxonomy is useful for TAM calculation.
Economic Impact Estimate
The economic impact estimate is trying to assess the value your
solution has for the customers and estimate how much they would be willing to
pay for that value. The basic logic is that if a particular product lowers your
cost by 10%, customers would be willing to pay, say, 1% of that cost to realize
that benefit. This rationale makes a lot of sense economically, however, I
consider this method rather unreliable.
First, companies are really mistrustful of any promise of hard
cost savings or revenue growth. All the ROI calculators in the world are
usually met with severe skepticism. On top of that, companies are very
reluctant to promise you a share of their savings or growth. What they want is a
predictable and elastic operating expense that they can throttle up and down as
needed. That’s why all the software is moving to the cloud – it shifts any risk
towards an operating expenditure.
Still, the TAM calculation based on the economic impact estimate
makes sense on a macro level. For example, you can estimate the impact of the
entire IT infrastructure on a particular sector – i.e. how much does technology
make a difference in banking or in retail. Or perhaps the impact of a major
industry trend such as mobility or IoT. But as you start getting more granular,
it’s hard to defend that your particular product has made all of the
contribution to the bottom line. That’s why using this methodology can lead to
unreasonable TAM estimates.
As you can see, there is more than one way to go and if you are
serious about estimating your TAM, I do recommend you try them all. Don’t
expect the results to be same but hopefully at least within the same number of
digits. If they differ by order of magnitudes, you might have to revisit some
of your estimates.
Good luck estimating and…trust your judgment!